www.industry-asia-pacific.com
01
'22
Written on Modified on
FRAUNHOFER ML4P PROJECT IMPROVES EFFICIENCY IN INDUSTRIAL MANUFACTURING
Artificial intelligence is regularly applied in areas such as image analysis and speech recognition. However, in the industrial production sector its potential is still scarcely used. Several Fraunhofer institutes have recently developed a solution as part of the lighthouse project “ML4P — Machine Learning for Production”, which aims to make industrial manufacturing much more efficient through the use of machine learning. The software suite is very flexible and can be easily applied in existing production processes.
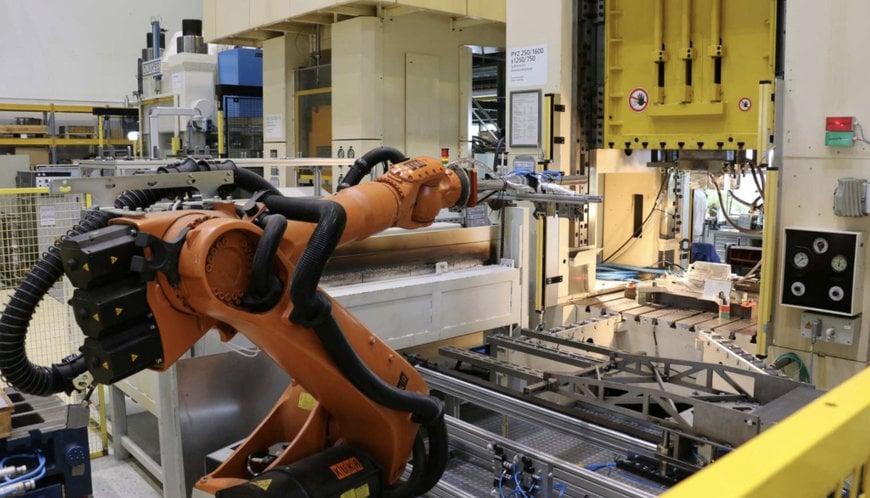
The production industry is a fundamental building block of the German economy. According to data from the German Federal Statistical Office, in 2017 there were more than 700,000 manufacturing companies with around 7.4 million employees producing a revenue of more than 2 billion euros. Companies from the automotive, electrical engineering, mechanical engineering, food production, plastics and chemical sectors all contribute to this economic strength. Many of these companies use large fleets of equipment and complex production facilities. Modern machines fitted with extensive sensors supply an increasing amount of data, resulting in huge optimization potential for production by means of data analysis using artificial intelligence (AI) and machine learning (ML).
A consortium comprised of several Fraunhofer institutes seeks to harness the previously untapped potential for the benefit of the industry. As part of the four-year lighthouse project “ML4P — Machine Learning for Production” lead by the Fraunhofer Institute of Optronics, System Technologies and Image Exploitation IOSB, the consortium has created an efficient solution based on ML technologies that companies can use to optimize their production processes. ML4P uses a combined approach consisting of a scientifically-based process model and software tools derived from this. The objective is to make production faster and more energy- and resource-efficient. The ML-based software suite can, for example, analyze machine data to discover hidden contexts and use these to optimize the manufacturing process. It is also capable of improving production on an ongoing basis thanks to its ability to learn. This is beneficial for the quality of products, too.
Multi-phase process model
However, the software is only one part of the ML4P approach — its process model acts as a key foundation. Christian Frey, Head of Systems for Measurement, Control and Diagnosis at Fraunhofer IOSB and ML4P project manager, says: “We don’t just pop up with a completed software solution for a company — instead, we guide them through the process model, taking a methodical, step-by-step approach.” The first step is to analyze the current state of the production process. Based on the results, the experts identify potential areas for optimization, set targets and develop a concept for implementing ML4P. In the next step, they examine whether the concept can actually be implemented with the available machinery and their data and how it lines up with the company’s objectives.
“The process model is divided into several phases which build upon one another. The decision as to whether a company actually opts for ML4P will only be taken when it is certain that the concept is viable, is easy to implement and economically reasonable,” says Lars Wessels, ML4P deputy project manager.
The following step involves transforming process data from the machinery into a comprehensive, digital information model. In this process, expert knowledge is just as important as the data. Engineers are actively involved and contribute their knowledge about all steps of the process, including the specific tasks and interactions of the machines. The expert knowledge is integrated into the ML4P pipeline, which learns a process model from machine data. Implementation and test operations follow afterwards. Eventually, the process model is deployed and daily production begins.
Flexible tools and industry standards
The software suite offers a range of tools for implementing ML-optimized production, including generic tools for typical tasks such as monitoring a machine’s operating state. These are compatible with a number of industrial communication interfaces such as OPC UA (Open Platform Communications Unified Architecture). Wherever possible, Fraunhofer researchers tried to avoid using proprietary software protocols, relying instead on established standards and programming interfaces.
Other strengths of this concept are its scalability and flexibility. Once put into operation, each module can be customized at any time and use the incoming new data to continuously update the process model and thus point out potential for further optimization. New machinery can be integrated as can most older machines — even those which are 30 or 40 years old.“ It is not so much about the machines, but whether it can provide suitable data, for example if it is equipped with dedicated sensors,” says Wessels. Smaller companies can also apply ML4P, even if they only want to optimize specific parts of a manufacturing process.
“Many companies are still skeptical about the use of artificial intelligence or ML because they have not yet recognized the enormous potential that machine learning offers for production. However, the modular platform from Fraunhofer provides transparency, flexibility and scalability, thus reducing the barrier to entry,” says Frey.
The ML4P team has already tested the integrated concept across various fields of application. Solutions for hot sheet metal forming were developed at the Fraunhofer Institute for Machine Tools and Forming Technology IWU. The Fraunhofer Institute for Factory Operation and Automation IFF has optimized the production of membrane filters and the Fraunhofer Institute for Mechanics of Materials IWM has tested the software suite at a glass-bending plant. The software tools were continuously improved based on the experience gained during these field tests.
“We are very happy that the ambitious ML4P project has been successfully completed after four years of work. For the first time, companies in the manufacturing business have the opportunity to experience the full optimization potential that machine learning can bring to production,” says Frey.
www.fraunhofer.com