AI is a journey, not a destination – Toshiba’s AI progresses to the next stage-Machine Learning Operations (MLOps) concept that automates the AI learning cycle
Smartphone apps are vital to our everyday lives. If you set them to update automatically, they will continue to be easy to use. AI (Artificial Intelligence) has also become a familiar presence, playing an active role in many aspects of our lives, from predicting product sales to recognizing people through a camera lense.
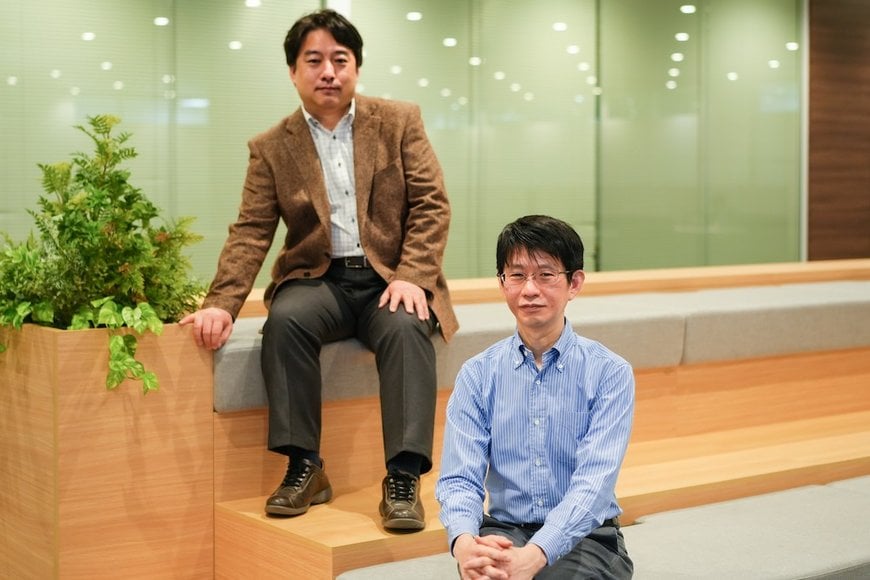
- AI is continuously evolving. What is our responsibility toward AI implemented in society?
- MLOps—automating the AI learning cycle that used to rely on humans.
- Accelerated verification of AI ideas through MLOps and its implications.
However, its performance is not maintained or improved automatically. The reality is that it relies on people. This will gradually lead to undesirable outcomes such as incorrect predictions and false recognition. Machine Learning Operations (MLOps) helps to solve these issues and makes AI a meaningful and effective tool for all of us. We met with some cutting-edge AI researchers who gave us a simple explanation of how MLOps works and the social implementation of AI that they seek to achieve.
Once applied, AI’s benefits become apparent, although that is also when we need a responsible approach
Toshiba has over fifty years of history in AI research and development. Our total number of patent applications in the field is third in the world, after IBM and Microsoft.* Now, after basic research, we are moving one step further to the stage of how to ensure quality that warrants enduring trust. This will enable us to fulfill the social responsibility of AI, not just create AI. Hopes are high for the MLOps platform in this regard. It brings together staff in charge of developing and operating AI, and provides seamless management from AI implementation to operation. Toshiba uses MLOps to maintain and improve the performance of AI even after it is made.
*WIPO Technology Trends 2019, issued by the World Intellectual Property Organization
Masataka Yamada leads the MLOps development project at the Corporate Research & Development Center, Toshiba Corporation, along with the Digital Innovation Technology Center that promotes Toshiba’s digital transformation. This cutting-edge AI research is going from “ready” to “take off,” so to speak. Yamada explains further:
“Toshiba is prepared to implement AI in our society and provide meaningful solutions. While we will of course continue to hone our AI technologies, we have already reached a satisfactory level in areas such as image recognition of post addresses and numbers. In addition, we are also building up technologies in sound recognition, data analysis and prediction, and other fields, which have started to bloom in many directions,” says Yamada.
Masataka Yamada, Expert, AI Platform Technology Dept., Advanced Intelligent Systems Technology Center,
Corporate Research & Development Center, Toshiba Corporation
It’s easy to imagine that AI will become more and more necessary to our society with trends such as the spread of autonomous driving. However, AI is made by “learning” from data. This means that you have to update the AI by giving it the latest data, otherwise you won’t be able to create meaningful solutions. “The true essence of machine learning comes after its implementation,” says Yamada, astutely pointing out the current challenge.
“AI delivers its true value when it is continuously maintained and improved. This also boosts its economic and social value. However, the current machine learning cycle still relies on humans. For example, it is humans who discover issues with AI. It’s also humans who improve them. The speed of improvement will not increase after implementation under these circumstances, and costs will jump back up.
This is where the MLOps platform steps up to the plate. If AI is developed and implemented on the back of MLOps, then the machine learning cycle will also be automated, and quality can be maintained. In other words, MLOps is what continuously maximizes the benefits we derive from AI,” says Yamada.
What image recognition leader Toshiba learned from its MLOps proof-of-concept tests
To sum up MLOps in a word, it’s a platform that smooths out the machine learning cycle. Development and operation of AI are carried out in harmony, and the performance of AI is continually maintained and improved. For example, one possible flow could be: (1) AI will provide inference services and people will benefit from them, (2) monitoring will detect unexpected abnormal data, and (3) AI will be able to relearn based on the data. Then, at the same time as returning to step (1) and making the inference service more in line with reality, (4) store newly acquired data and past AI models in the base of data. MLOps drives steps (1) to (4) automatically. Just as the name suggests, it smooths out the operations of the machine learning cycle.
How Machine Learning Operations (MLOps) automatically and continually maintains and improves AI performance.
Toshiba is moving forward to proof-of-concept tests in order to make MLOps a standard platform. One of these tests was carried out in the Toshiba’s cafeteria for two weeks from July 15, 2021, by Yamada’s Corporate Research & Development Center and the aforementioned Digital Innovation Technology Center. In this test, image recognition AI detected users of the cafeteria and measured the flow and density of people. The purpose of the test was to apply MLOps to monitor the accuracy of AI inference and verify whether an alert would be triggered if results deviating from the norm continued to occur. To this end, the team deliberately caused problems such as backlighting, halos, obstacles, and communication noise to thoroughly verify whether the MLOps monitoring functioned sufficiently and to what extent the cycle could be automated.
Overview of MLOps verification test
The most significant result of this test was that MLOps was not only able to automate alerting of anomaly detection, but also AI relearning. We asked Masayuki Maruyama, an image recognition expert from the Corporate Research & Development Center, Toshiba Corporation, who has been developing MLOps PoC* since 2021 to explain the test in his own words.
*Proof of Concept: Verifying and confirming the feasibility of a new concept or idea.
“Image recognition is a field in which unexpected aspects often affect AI algorithms. In other words, AI’s bias toward making false judgements in response to noise is particularly striking. For this reason, it was important in this test to make sure that MLOps could correctly identify noise as noise and use it in relearning for the AI. It was also one of the difficult aspects of the test,” says Maruyama.
Masayuki Maruyama, Specialist, AI Application Dept., Advanced Intelligent Systems Technology Center,
Corporate Research & Development Center, Toshiba Corporation
To put it another way, when using AI, it is important to separate the cause of noise from whether it is an affect of the external environment or a problem on the AI side. Even when AI technicians would be able to make a judgement right away, it would take more time and effort than necessary to respond based on user-supplied information. What’s more, even thoroughly verified AI algorithms often collect unexpected data in real-world environments. There would never be enough staff to cover for going to the site every time that happens and making the AI relearn.
“It is very meaningful that we were able to confirm that the MLOps platform was able to handle everything up to automating relearning in this test. This is because it can notice immediately and make corrections immediately. In particular, we have made progress in reducing the workload of AI technicians especially from the standpoint of promoting the verification of the feasibility of ideas,” says Maruyama.
Not to be forgotten, MLOps’ data management infrastructure!
Another important point is that Toshiba’s MLOps has demonstrated potential to be a data management infrastructure that optimizes AI development. This is because in the previously mentioned proof-of-concept test, it was able to ensure the reproducibility of the data, including the differences from the last time it was trained, the specifications of the data, and the version control of the AI. MLOps, which has moved into the territory of data management infrastructure, could be called a cutting-edge initiative on a global level.
This means that the elimination of all challenges that could happen after implementing AI is now in sight. For example, the characteristics of data for AI to infer may change without warning with the passage of time, and accuracy may decline. If a data management infrastructure is in place at times like these, it will be clear at which point the characteristics of the data changed, and AI algorithms can be swiftly made to relearn. Noise and precision monitoring were challenges in keeping AI precision from falling. MLOps, with its data management infrastructure function, goes one step further.
“The fact that technicians shared insights through MLOps in this test made me feel that we have a bright prospect. If the function of the data management infrastructure improves, it should be possible to greatly reduce the burden of verifying ideas in terms of time, cost, and labor. MLOps will usher in a new stage for the social implementation of Toshiba’s AI, and progress will accelerate across all fields,” says Maruyama.
Run the learning cycle according to the AI implementation environment
The proof-of-concept test was carried out in an on-premises environment (AI implemented not in an internet cloud but in a user facility), but Toshiba naturally envisages the use of AI in a cloud environment. Both have their pros and cons. While cloud environments boast high processing capabilities, downsides such as delays in telecommunications can be anticipated. Some people have also raised concerns about the security risk of processing data including personal information. In on-premise environments, on the other hand, data is processed on site which enables a high-level of real-time capabilities and robust protection of personal information, but costs are an issue when it comes to advanced data processing. So, how about the MLOps platform that promotes the AI learning cycle?
“I’m thinking that the AI implementation environment will be chosen on a case-by-case basis, whether it be on-premises or cloud—in other words, on-site or via the internet. In this regard, MLOps itself does not regulate AI algorithms but just manages the procedure for learning, so its functionality does not rely on infrastructure. What’s more, it is structured so as not to rely on a particular cloud, and it can manage one AI in an on-premises environment or on the cloud,” says Yamada.
Anticipations are high for AI to be a means of changing the way business and society works. That’s why Toshiba is trying to break new ground in AI across the organization. For example, discussions have started on making guidelines to standardize AI quality. The MLOps has also seen between technicians and departments deepen collaboration and work hard together in other fields such as IoT and sensors where the method of obtaining data is key.
MLOps is a platform that brings new solutions to optimizing the AI learning cycle. It integrates the expertise of Toshiba, which has evolved in tandem with society over the course of its approximately 150 years of history. There is no doubt that a “future of AI” awaits us—one where we develop AI that is needed by society whilst fulfilling our social responsibility, including ensuring its continued quality.
www.toshiba.com